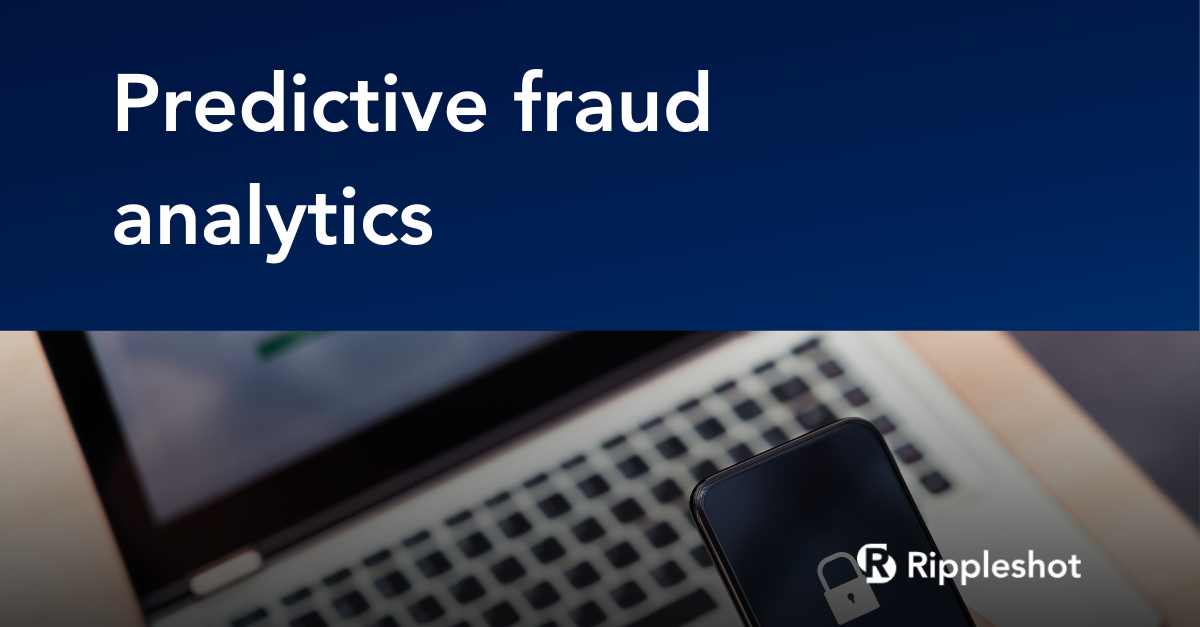
Predictive Fraud Analytics: The Key to Proactive Risk Management
Fraud is advancing at an alarming rate, leaving financial institutions struggling to keep pace. As fraudsters develop more sophisticated tactics, institutions are faced with a critical challenge: how to stop these attacks before they cause serious damage.
For financial institutions like credit unions, community and regional banks, there is an increasing need to utilize large data sets and analytics to identify, track and stop fraud. Speed and size matter. That’s where predictive fraud analytics comes into play – offering a proactive and large model data-driven approach to fraud risk management that empowers institutions to detect and stop fraud before it occurs.
The Need for Predictive Fraud Analytics
Fraud is getting more complex by the day. Criminals are using advanced techniques, like creating synthetic identities and exploiting weaknesses in digital transactions, to bypass traditional detection methods. Even insider threats – where employees misuse their access – have become a growing concern.
Traditional fraud detection systems often rely on set rules, catching fraud only after it occurs. By then, the damage is done. The rising complexity of these threats has made it clear: financial institutions must shift from reactive to proactive strategies.
Powered by AI and machine learning, predictive fraud analytics is a pivotal solution that allows institutions to stay one step ahead of fraudsters’ cunning ways, helping them to identify suspicious activity before it escalates into a full-blown attack.
How Predictive Fraud Analytics Works
At its core, predictive fraud analytics leverages advanced machine learning algorithms to detect patterns and anomalies across vast amounts of data. By analyzing transaction histories, fraud patterns, and behavioral anomalies, these models can identify suspicious activity to allow the financial institution to take action in real-time. The key strength of predictive fraud analytics lies in its ability to learn and adapt over time. Unlike traditional systems, which are often hardcoded, predictive models continuously evolve, learning from each new data point to improve detection accuracy.
Using vast datasets that include transactional data and historical fraud activities, predictive fraud analytics creates models capable of pinpointing irregular activities with precision. These models don’t just react to known fraud patterns – they proactively detect emerging schemes, providing early warnings that allow financial institutions to mitigate risk before fraud can occur.
Key Benefits of Predictive Fraud Analytics for Financial Institutions
Implementing predictive fraud analytics offers a range of advantages that go beyond traditional fraud detection methods:
1. Proactive Risk Management
The biggest advantage is moving from a reactive to a proactive stance. Instead of relying solely on systems that detect fraud after it occurs and then pulling in team members and hours of work to fix the damage, institutions can predict and prevent fraud before it strikes, reducing risk and keeping fraudsters at bay.
2. Fewer False Positives
One of the biggest frustrations with traditional fraud detection is the high number of false positives. Legitimate transactions often get flagged as suspicious, frustrating customers and wasting valuable resources. Predictive models are much better at reducing these false alarms, allowing fraud teams to focus on real threats and avoid unnecessary disruptions to customers. The result, increased card holder experience and trust.
3. Faster Fraud Detection and Response
Timing is critical when it comes to fraud detection. The faster you can catch fraud, the less damage is done. With predictive fraud analytics, financial institutions can proactively take action in real-time – drastically cutting down detection and response times. This means fraud is caught before it spirals out of control.
4. Scalable and Adaptable
As financial institutions grow, so do their transaction volumes. Traditional fraud detection systems can quickly get overwhelmed by the sheer volume of data, but predictive fraud analytics is built to scale. Whether it’s handling thousands or millions of transactions, these systems can easily adapt and keep up with new types of fraud as they emerge.
Real-World Use Cases: Predictive Fraud Analytics in Action
Financial institutions, such as JPMorgan Chase, are successfully using predictive fraud analytics to detect and prevent fraudulent transactions in real-time. By leveraging AI and machine learning algorithms, JPMorgan Chase’s system processes vast amounts of transaction data to identify suspicious patterns, enabling immediate responses and minimizing the impact of fraud on customers.
The system continuously evolves with each transaction, enhancing its detection capabilities over time. This proactive approach has significantly reduced fraud incidents and financial losses for the bank – all thanks to their use of predictive fraud analytics.
Today, technology is the great leveler: at Rippleshot, we believe there’s no reason why every community and regional bank and credit union should not have access to this same level of technology.
The Future of Fraud Prevention
Looking ahead, the battle against fraud isn’t going to get any easier. Fraudsters are constantly refining their techniques, which means financial institutions need to be just as agile. Fortunately, advancements in AI and machine learning are making predictive fraud analytics even more effective. As models continue to evolve and learn from new data, financial institutions will be better equipped to fight off emerging threats.
The takeaway? Financial institutions that adopt predictive fraud analytics now will be well-positioned to face the future of fraud prevention, while those who stick with reactive methods may find themselves struggling to keep up.
Stay Ahead with Predictive Fraud Analytics
The future of fraud prevention is clear: institutions that want to stay ahead of fraud tactics must adopt a proactive approach. With predictive fraud analytics, financial institutions can transform their fraud detection strategies, catching fraudulent activity before it causes irreversible damage.
Now is the time to rethink your fraud prevention strategy. Adopting predictive fraud analytics isn’t just about staying competitive – it’s about protecting your institution and your customers from tomorrow’s threats.
Schedule a product tour with Rippleshot today to discover how we can help you lead the charge in proactive fraud management, reduce losses, and enhance operational efficiency.
Request a Product Tour
You have fraud frustrations? We have the solutions. Let's discuss what you are dealing with and we can learn more and share how we can help.